Forests offer a home to much of the world’s diverse array of plants and animals and provide essential natural resources from timber and food to medicinal plants. Despite the key role forests play in the world’s environmental and economic health, we continue to lose forests, along with the endangered animals that live in them. Accurate mapping of forest composition is of great importance for a sustainable management of forest ecosystems since information on tree species distribution can affect the exploitation and management policies of forests.
Over the last 40 years, extensive research has been devoted to the use of remote sensing data for tree species classification. Compared with traditional field surveys, remote sensing is one of the technological tools at our disposal: it decreases the cost of data acquisition, increases the area it is possible to cover without sampling, and enables the production of high-resolution forest maps.
To obtain better classification accuracy of tree species, many researchers combine multiple remote sensing data sources and methods to classify tree species. However, one of the key issues is automatic and precise classification methods for tree species mapping.
The commonly used methods include traditional machine learning methods such as support vector machine (SVM), extreme gradient boosting (XGB), random forest (RF), and fuzzy mathematics. Although many adopted methods of tree species classification have achieved satisfactory accuracy results, there is still a lack of highly automated and robust classification algorithms with high classification accuracy.
Based on field survey data of tree species in the northeast of China, a study led by Prof. REN Chunying from the Northeast the Institute of Geography and Agroecology, Chinese Academy of Sciences mapped the detailed tree species distribution by multiple machine learning algorithms in Jianshe forest farm.
To explore which machine learning (ML) algorithms can effectively extract various information of images to achieve higher accuracy, five ML algorithms were compared to identify the composition of tree species with multitemporal Sentinel-2 images in the Jianshe forest farm, Northeast China.
Three major types of deep neural networks (Conv1D, AlexNet, and long short-term memory) were tested to classify Sentinel-2 time series, which represent three disparate but effective strategies to apply sequential data. The other two models are support vector machine (SVM) and random forest (RF), which are renowned for extensive adoption and high performance for various remote sensing applications.
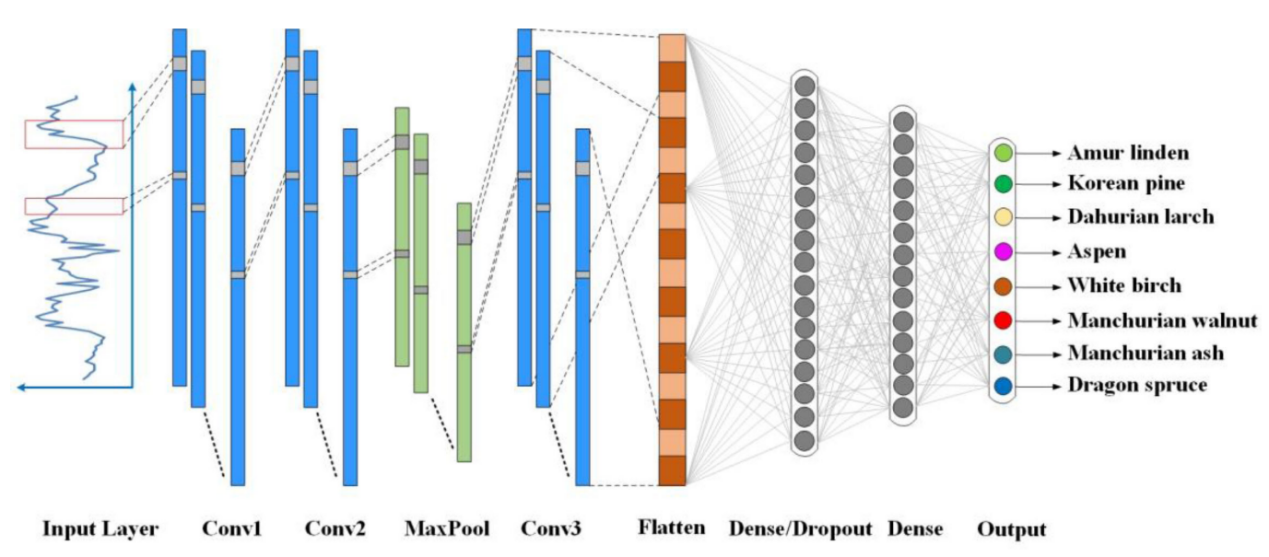
Figure 1 One-dimensional convolutional neural network model
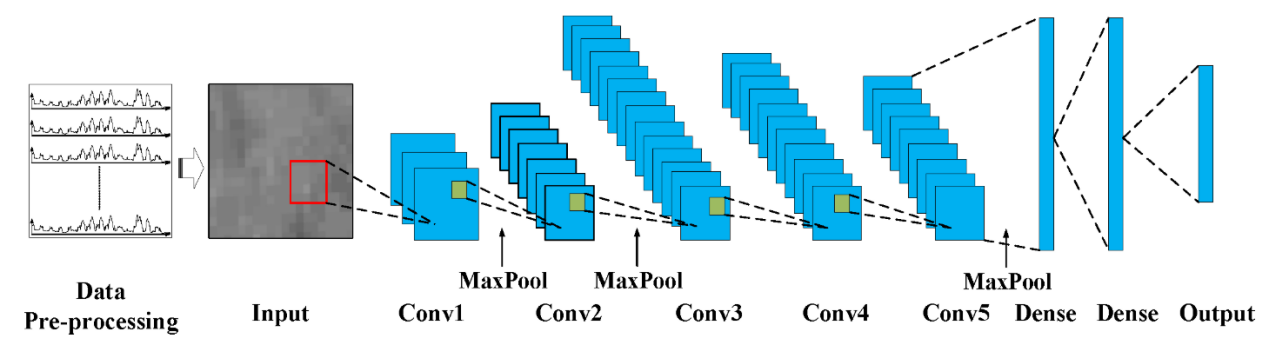
Figure 2 Architecture of the AlexNet model
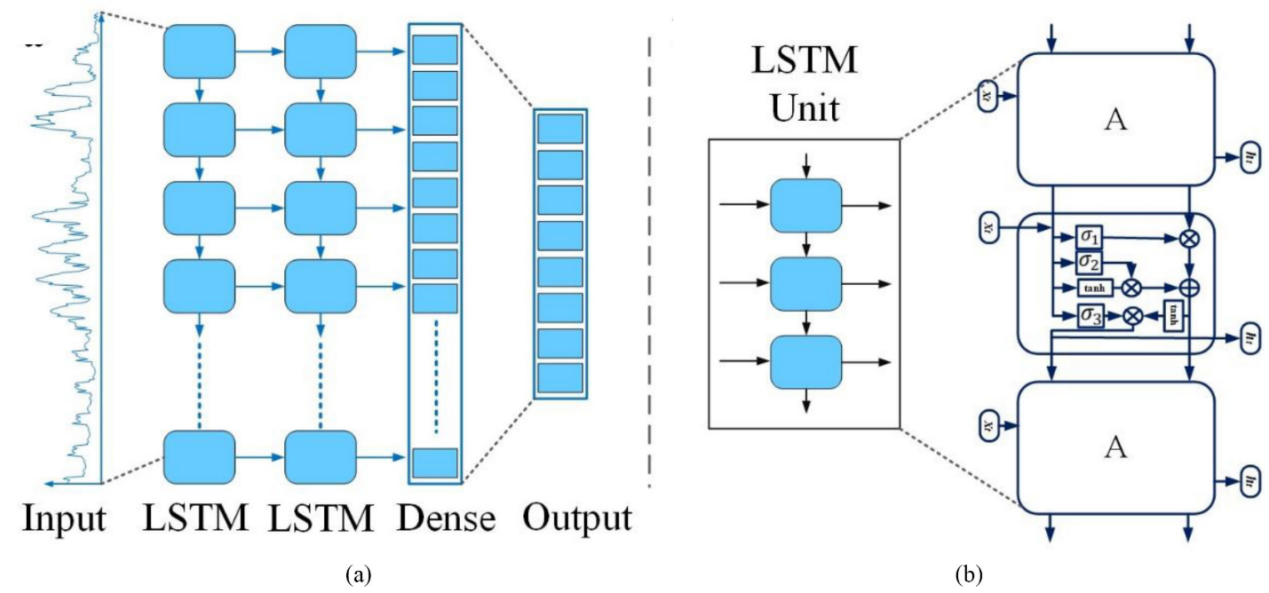
Figure 3 (a) Architecture of LSTM model, (b) each LSTM unit.
The results show that the overall accuracy of neural network models is better than that of SVM and RF. The Conv1D model had the highest classification accuracy (84.19%), followed by the LSTM model (81.52%), and the AlexNet model (76.02%). For non-neural network models, RF’s classification accuracy (79.04%) is higher than that of SVM (72.79%), but lower than that of Conv1D and LSTM. In addition, this study also selected the most suitable spectral and phase features for classification by using mean decrease in accuracy.
Specifically, the most important bands are the Short-wave infrared band and the red band, followed by the red-edge band. For the time phase features, images from May, June, and July are more useful compared to those from late summer and autumn. In general, this research shows that, among numerous alternative classification methods, deep neural network models combined with multitemporal Sentinel-2 images provide a new option for tree species mapping in future. The result was published on IEEE Journal of Selected Topics in Applied Earth Observations and Remote Sensing in 2021.
This work was supported in part by the National Key Research and Development project and in part by the National Natural Science Foundation of China.
Contact:
REN Chunying
Northeast the Institute of Geography and Agroecology
Tel: 0431-85542297
E-mail: renchy@iga.ac.cn